Gaussian Process interpolation using treegp¶
Quick start¶
Here is a simple example of a 2D gaussian process interpolation
using treegp
. Before showing how to use treegp
, the interpolation needs
to be perform over some data and we will simulate some Gaussian random field below.
In the block bellow you can find how:
How to generate a 2d anisotropic gaussian random field
How to write the correlation length matrix in terms of the isotropic correlation lenght and shear parameters (\(g_1\), \(g_1\)); see Léget et al 2021
import numpy as np
import treegp
from treegp import AnisotropicRBF
import matplotlib.pyplot as plt
def get_correlation_length_matrix(size, g1, g2):
"""
Produce correlation matrix to introduce anisotropy in kernel.
Used same parametrization as shape measurement in weak-lensing
because this is mathematicaly equivalent (anistropic kernel
will have an elliptical shape).
:param correlation_length: Correlation lenght of the kernel.
:param g1, g2: Shear applied to isotropic kernel.
"""
if abs(g1) > 1:
g1 = 0
if abs(g2) > 1:
g2 = 0
g = np.sqrt(g1**2 + g2**2)
q = (1 - g) / (1 + g)
phi = 0.5 * np.arctan2(g2, g1)
rot = np.array([[np.cos(phi), np.sin(phi)], [-np.sin(phi), np.cos(phi)]])
ell = np.array([[size**2, 0], [0, (size * q) ** 2]])
L = np.dot(rot.T, ell.dot(rot))
return L
def make_2d_grf(kernel, noise=None, seed=42, npoints=40):
"""
Function to generate a 2D gaussian random field for a
given scikit-learn kernel.
:param kernel: given sklearn kernel.
:param noise: float. Level of noise to add to the
gaussian randomn field. (default: None)
:param seed: int. seed of the random process. (default: 42)
:param npoints: int. number of points to generate for the
simulations.
"""
# fixing the seed
np.random.seed(seed)
# generate random 2D coordinate
x1 = np.random.uniform(-10, 10, npoints)
x2 = np.random.uniform(-10, 10, npoints)
x = np.array([x1, x2]).T
# creating the correlation matrix / kernel
K = kernel.__call__(x)
# generating gaussian random field
y = np.random.multivariate_normal(np.zeros(npoints), K)
if noise is not None:
# adding noise
y += np.random.normal(scale=noise, size=npoints)
y_err = np.ones_like(y) * noise
return x, y, y_err
else:
return x, y, None
L = get_correlation_length_matrix(2., 0.3, 0.3)
invL = np.linalg.inv(L)
kernel = 2**2 * AnisotropicRBF(invLam=invL)
x, y, y_err = make_2d_grf(kernel, noise=0.1, seed=42, npoints=2000)
plt.scatter(x[:,0], x[:,1], c=y, cmap=plt.cm.seismic, vmin=-4, vmax=4)
cb = plt.colorbar()
cb.set_label('y')
plt.xlabel('$x_0$')
plt.ylabel('$x_1$')
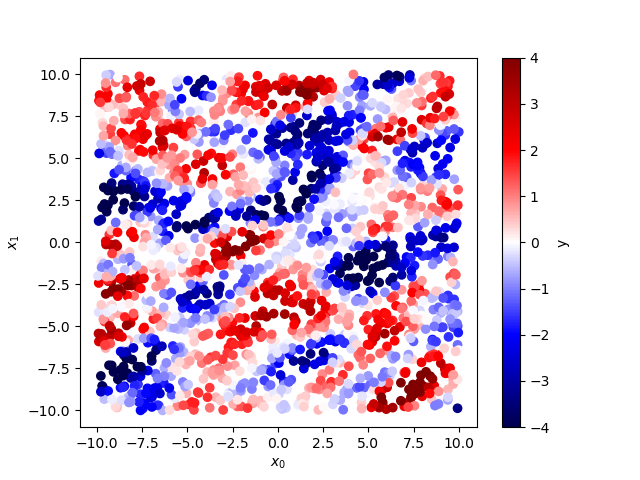
To do the gaussian process interpolation with treegp
it follow this API:
kernel_treegp = "2**2 * AnisotropicRBF(invLam={0!r})".format(invL)
gp = treegp.GPInterpolation(
kernel=kernel_treegp,
optimizer="anisotropic",
normalize=True,
nbins=21,
min_sep=0.0,
max_sep=1.0,
p0=[6., 0.0, 0.0],
)
# feed with data treegp
gp.initialize(x, y, y_err=y_err)
# solve for hyperparameters
gp.solve()
# test on test position and compute GP interpolation on it.
x1_test = np.random.uniform(-10, 10, 1500)
x2_test = np.random.uniform(-10, 10, 1500)
x_test = np.array([x1_test, x2_test]).T
y_test, y_test_cov = gp.predict(x_test, return_cov=True)
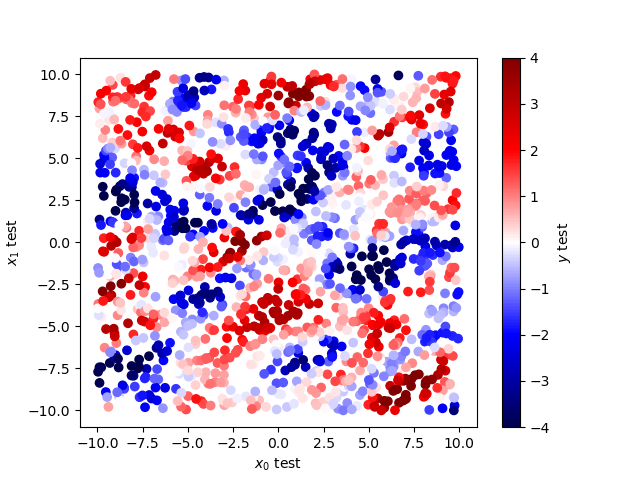